As AI applications continue to evolve, the demand for powerful GPUs for machine learning and deep learning training has grown exponentially. While NVIDIA has dominated the GPU market, AMD GPUs for AI are gaining traction, offering strong performance at a competitive price point. In this article, we’ll delve into the capabilities of AMD GPUs, focusing on the AMD ROCm platform, TensorFlow and PyTorch compatibility, and how AMD compares to NVIDIA in AI workloads.
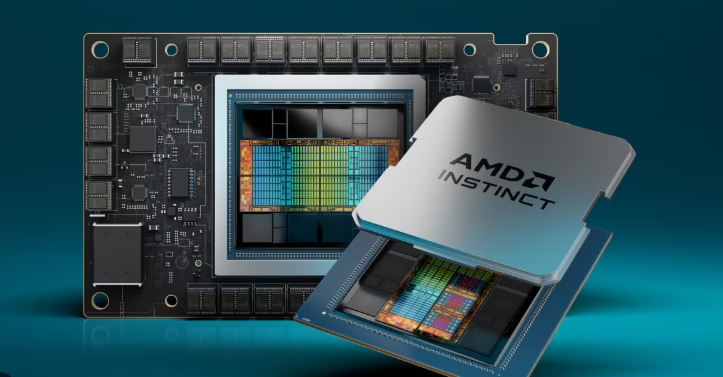
Table of Contents
ToggleCan an AMD GPU be Used for AI?
Absolutely. AMD GPUs are capable of powering AI workloads, especially with the support of their ROCm (Radeon Open Compute) platform. ROCm enables AMD GPUs to run machine learning frameworks like TensorFlow AMD GPU and PyTorch AMD GPU, providing an efficient alternative to NVIDIA’s CUDA ecosystem. The compatibility of AMD GPUs with these popular frameworks has significantly improved, making them a viable option for AI training.
Which AMD GPU is Best for AI?
When it comes to the best AMD GPU for AI/Deep learning, the AMD RX 7900 XTX and AMD Instinct MI325X are often top contenders. If you are looking to puechase AMD GPU for Ai, Please contact to get quote. Featuring 2 AI Accelerators per compute unit – Radeon RX 7000 Series Graphics cards deliver up to 123 TFLOPs of FP16 performance. Whats more, the AMD MI325X OAM (256 GB) achieves up to 1.3 times the AI performance compared to the NVIDIA H200 SXM (141 GB), showcasing its strength across all tested precision levels. These GPUs are specifically designed for data centers and AI tasks, delivering exceptional performance in AI training and deep learning applications. The MI325X, in particular, is known for its high-bandwidth memory and parallel processing capabilities, which are critical for intensive AI workloads.
AMD ROCm: The Backbone of AI Performance
The AMD ROCm platform is at the core of AMD’s AI capabilities. It provides a robust open-source framework that supports various AI and machine learning workloads. ROCm offers support for TensorFlow, PyTorch, and other frameworks, enabling developers to harness the power of AMD GPUs for AI without being locked into NVIDIA’s ecosystem. This flexibility is particularly appealing for researchers and enterprises seeking cost-effective alternatives.
TensorFlow AMD GPU and PyTorch AMD GPU: Full Compatibility
Both TensorFlow AMD GPU and PyTorch AMD GPU are optimized to run on AMD GPUs with the help of ROCm. While these frameworks have historically been more NVIDIA-centric, the improvements made to ROCm have significantly enhanced AMD’s AI training capabilities. Users can now train complex models on AMD GPUs, ensuring high performance without the premium price tag often associated with NVIDIA products.
AMD GPU for AI Price: A Competitive Edge
One of the key advantages of choosing an AMD GPU for AI is the price. AMD GPUs typically come at a lower cost than their NVIDIA counterparts, offering great performance per dollar. This makes them an attractive option for developers and researchers who need powerful AI hardware but are working within a budget.
Can AMD Beat NVIDIA in AI?
While NVIDIA GPUs are still considered the industry standard for AI workloads, AMD is catching up quickly. With advancements in ROCm, support for popular frameworks like TensorFlow and PyTorch, and GPUs tailored for AI, AMD has positioned itself as a worthy competitor in the AI space. Whether AMD can completely beat NVIDIA in AI remains to be seen, but for those seeking a cost-effective solution, AMD is certainly a strong alternative.
Conclusion
AMD’s GPUs are rapidly becoming a powerful tool for AI training. With the support of the ROCm platform, compatibility with frameworks like TensorFlow AMD GPU and PyTorch AMD GPU, and competitive pricing, AMD GPUs for AI are an excellent option for those looking to harness the power of artificial intelligence without breaking the bank. If you’re exploring alternatives to NVIDIA, the best AMD GPU for AI might just be the performance and value solution you need.